Selector returned to Networking Field Day this year to present our latest developments in network AIOps. Cofounder and CTO Nitin Kumar, along with VP Solutions Engineering Debashis Mohanty and Principal Solution Architect John Heintz, explored how Selector’s GenAI-driven conversational interface promises to not only address today’s network operations challenges, but transform the industry.
Read on to catch the highlights of the live-streamed presentation which occurred on July 11, 2024.
Today’s Network Operations Challenges
The scale and complexity of modern networks has made it increasingly difficult to make sense of the available data and support incident remediation workflows. For instance, if cash registers at a retail establishment cannot connect to the payment application, the issue could reside with a number of different elements within the stack, including the wifi, local area network (LAN), software-defined wide area network (SD-WAN), Internet, multiprotocol label switching (MPLS), cloud services (e.g., AWS, Azure), or even the payment application itself (Figure 1). Experts or vendors for each of these domains may have access to the associated data, but the average user cannot collect all the data nor holistically interpret it.
The ability to access the data and associated insights from all of these domains is known as data democratization. Selector supports data democratization through our GenAI-powered conversational interface as well as our consolidated smart alerting—both available via the customers’ preferred communication platform.
Bridging The Chasm
Obstacles currently exist which prevent the application of open-source AI services (e.g., ChatGPT, Microsoft Copilot, Google Gemini) to data originating from network infrastructure. To start, there’s the logistical issue of transporting the on-premises network operations data to these predominantly cloud-based services. The raw data is not only spread across thousands of devices, but often originates from different domains, vendors, and protocols. Further, the data exists in multiple formats, such as metrics, logs, events, and topology, and the accompanying metadata may be inconsistent and malformed.
Additionally, open-source AI services are trained in English—not the nuanced semantics of network operations data. And lastly, these AI services mine your data and pass it along to other organizations, presenting a significant security and privacy risk.
Selector solves these issues, and effectively bridges the chasm, through our innovative approach to data collection, processing, and analytics, in conjunction with application of precisely trained LLMs (large language models).
Mapping English Phrases to Queries
Selector’s processing of English phrases and subsequent application of GenAI relies on two core features. The first one involves addition of a uniform query interface to the data storage layer. For this interface, Selector chose SQL (structured query language). The second feature is LLM-translation of the English phrase to a SQL query so that it can access the query interface.
The diagram below (Figure 1) depicts how Selector leverages our LLM to translate an English phrase into a query, as seen in the translation layer. The returned query then runs on the query layer, which exists above the data storage layer.
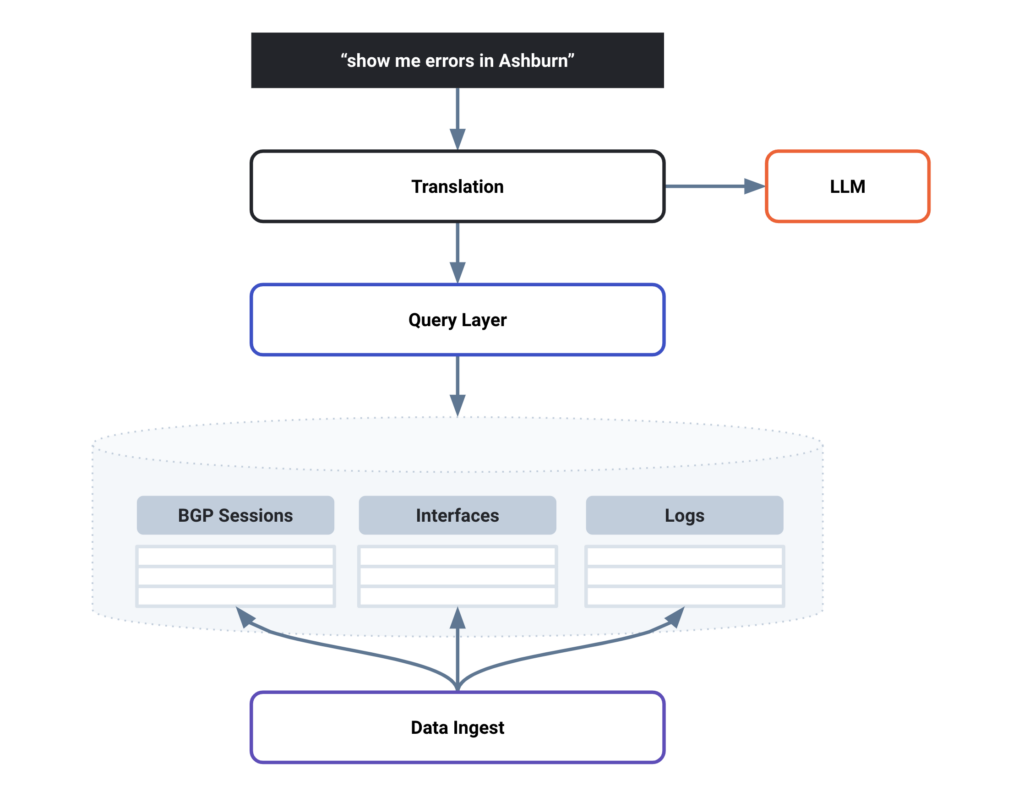
Inside the Selector LLM
The Selector LLM first determines which table or tables to query. Then, it focuses on keywords (e.g., Ashburn, GigE100*, last 2 days), applying these as filters to the table.
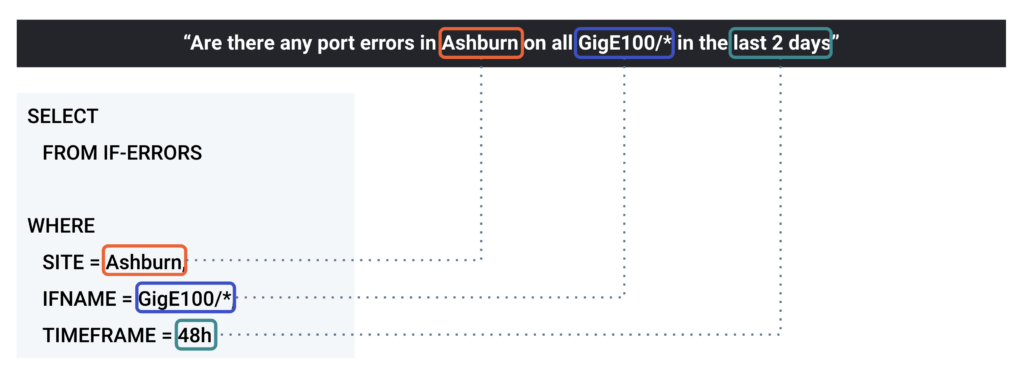
The LLM manages these tasks through a process known as imputing, or inferring (Figure 3). A typical function takes input and computes output using a set of parameters. An LLM takes output and using a model, imputes a function and its parameters—a manner of reverse mapping.
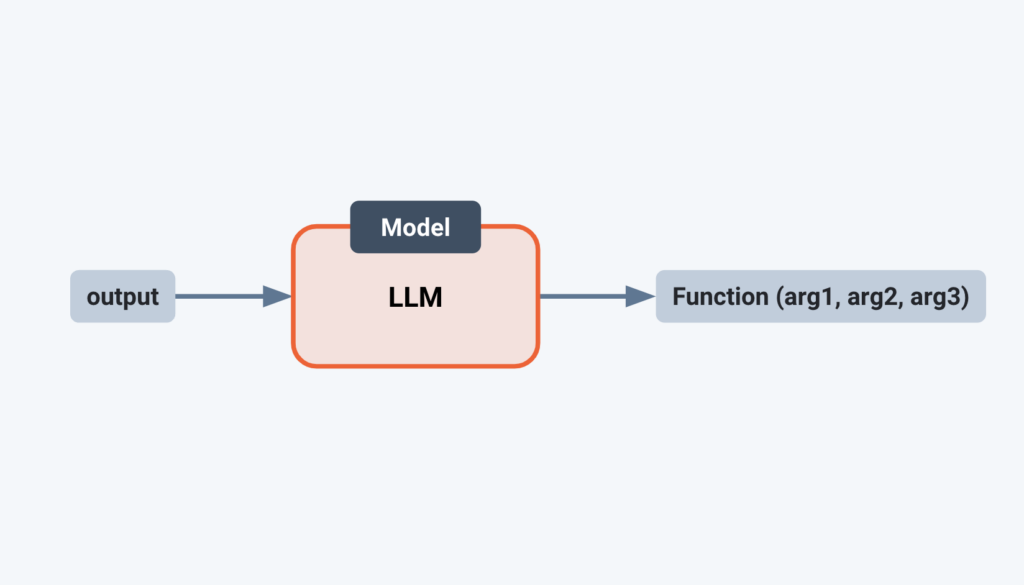
At its core, the Selector LLM model relies on a base, open-source model from the public domain. From there, it is trained with English data, enabling it to understand English language phrases. Next, Selector fine-tunes the LLM model with a dataset that teaches it to convert from English to Selector’s SQL query interface. These two steps do not involve the customer and occur in Selector’s cloud. We publish and deploy updates to this model periodically.
Then, for each customer deployment, Selector fine-tunes the LLM model with client-specific data and entities—a process which occurs on-premises at the customer. In this way, models are customized to each client’s unique solution. For example, Customer A’s model is different from Customer B’s and Customer C’s (Figure 4). The models are atomic and can be on-premises or in the cloud, depending on the client’s preference.
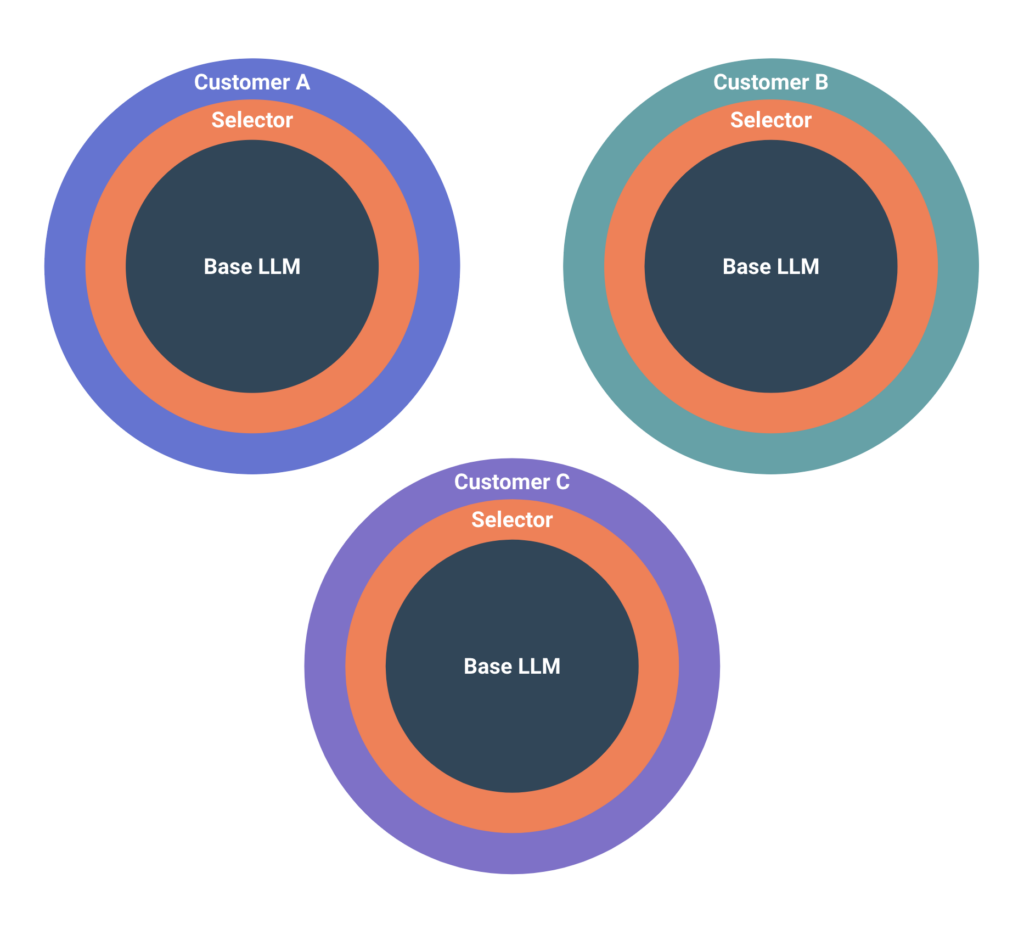
Selector Copilot
Selector Copilot, our GenAI-driven conversational interface, enables network operators to ask questions in plain English. For example, an operator might prompt Selector Copilot to “Show me errors in Ashburn” or pose the question, “Are there any port errors in Ashburn on all GigE100* in the last 2 days?”
For each query, Selector Copilot returns a visualization and summary of the results. Users can then interact with Selector to explore these results. They can also copy and paste each visualization from Selector Copilot onto a dedicated dashboard.
As an example, let’s say a cloud service provider asks Selector Copilot about the latency of their transit routers over the last two days. Selector Copilot delivers a visualization and summary, revealing higher latency within a certain region. The user can then drill down into network topology information to further investigate the higher latency, accessing relevant details about devices, interfaces, sites, and circuits.
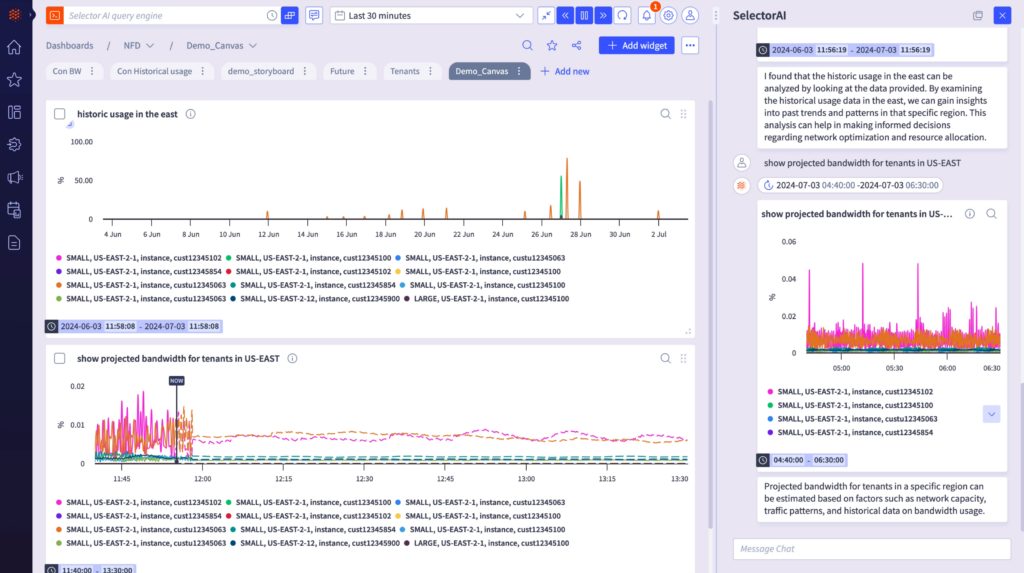
Selector Alerting
Selector’s consolidated alerts reveal the nature and severity of an incident along with the probable root cause and a comprehensive list of affected devices.
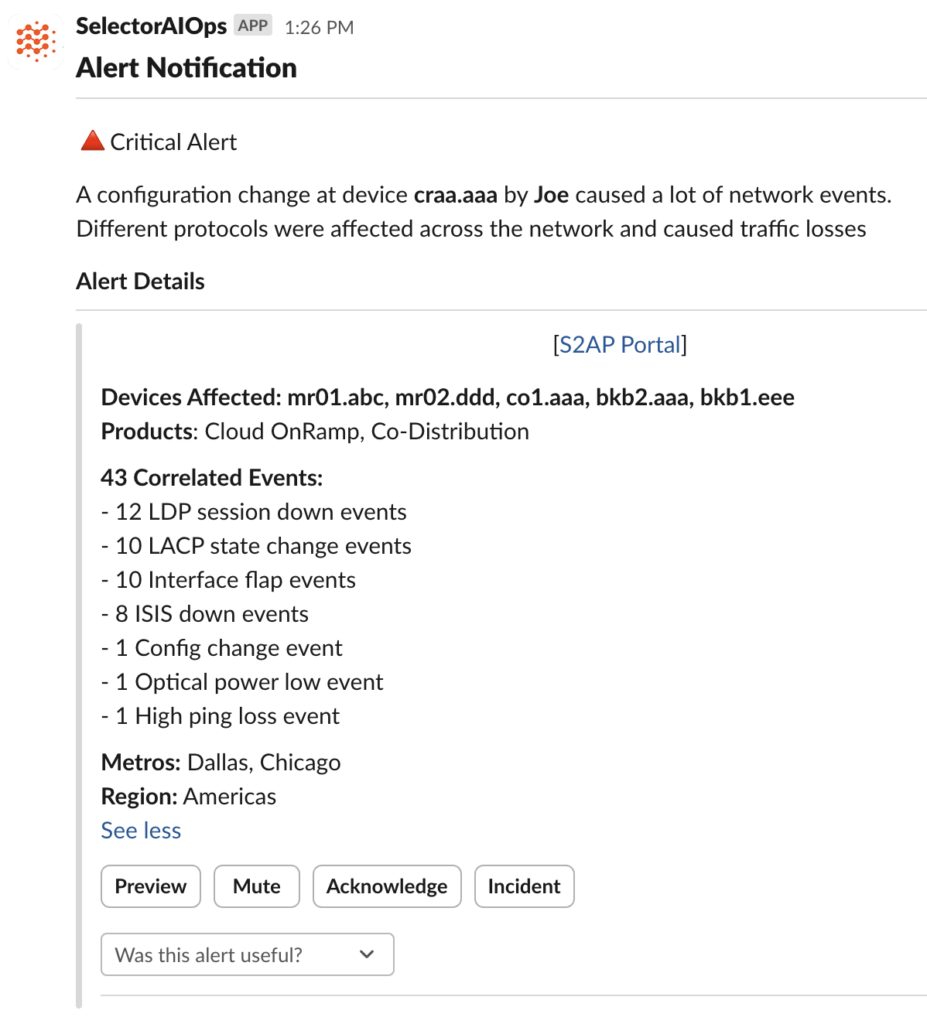
Selector’s alerting process relies on a pipeline in which machine learning and statistical algorithms first determine violations or anomalies across all network, application, and infrastructure data. These events are then clustered into correlation trees or graphs which indicate a single incident.
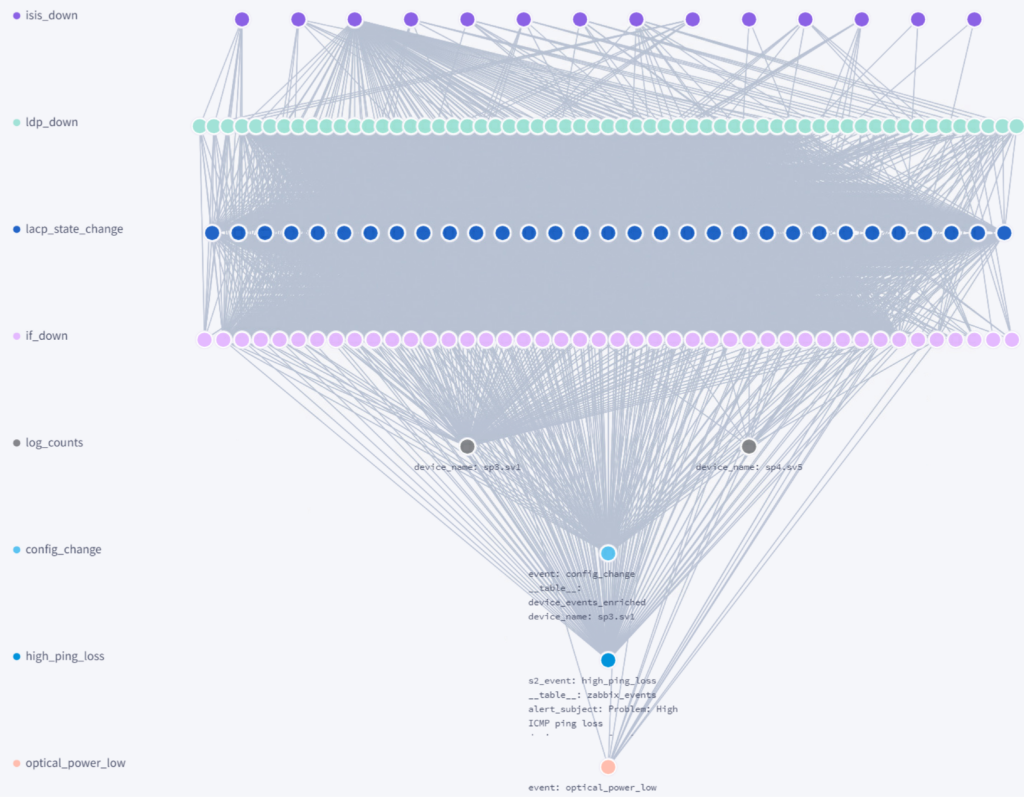
Selector summarizes these underlying event correlation graphs into consolidated alerts delivered via the customers’ preferred collaboration platform such as Slack or Microsoft Teams. Alerts can also be configured to automatically create tickets and map all relevant information into them. This enables downstream teams to commence remediation activities.