Picture this: Your phone rings in the middle of the night. It’s your engineering lead, calling to inform you of a significant outage affecting your customer-facing services. As your network operations team jumps into action, they’re greeted with chaos. Over 40 alerts flood their screens simultaneously. Your network, infrastructure monitoring, and application performance monitoring tools all fire independently, each with its own dashboard and presenting data in incompatible formats.
It’s like trying to solve a jigsaw puzzle blindfolded, with pieces scattered across multiple rooms and no map of how they connect. Without full-stack visibility across all layers, valuable time is lost trying to piece together the fragmented clues, which prolongs downtime and costs businesses thousands of dollars per minute. The longer it takes to identify the root cause, the longer your customers and revenue will remain impacted. In scenarios like these, disconnected data isn’t just inconvenient. It’s financially devastating.
Why Disconnected Data Is Holding Back Network Operations
Today’s enterprises are drowning in data but starving for insights. Operations teams, like in the example above, face the daunting challenge of managing massive volumes of telemetry from across the entire technology stack, spanning network hardware, infrastructure platforms, and distributed applications. Each system and vendor produces data in a different format, resulting in isolated information scattered across dozens of dashboards and tools. As data volumes surge, the task of troubleshooting becomes not only overwhelming but often nearly impossible.
At the core, this isn’t just a complexity problem. It’s a data quality problem. Before organizations can leverage advanced technology like Artificial Intelligence for IT Operations (AIOps), they must first confront a foundational yet often overlooked challenge: data harmonization.
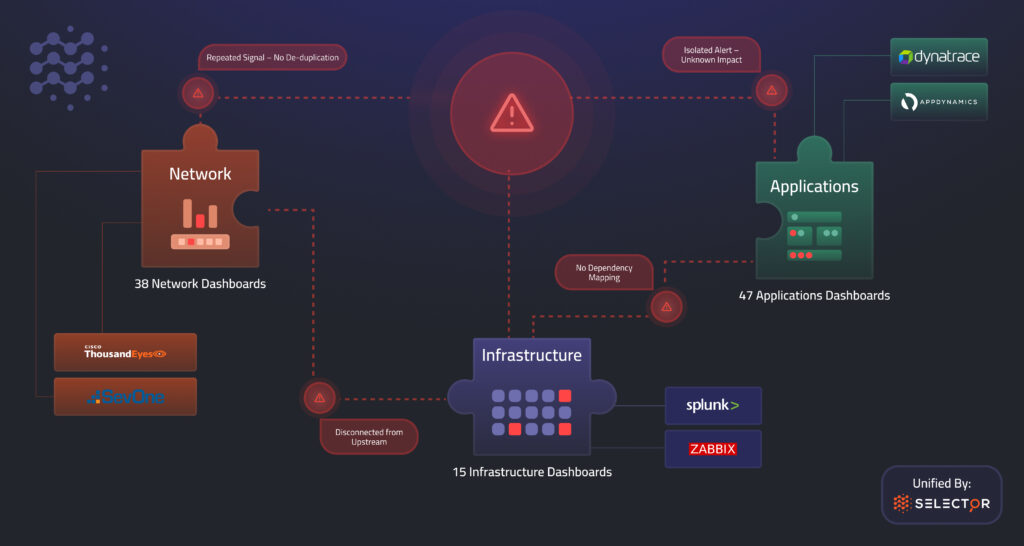
Introducing Selector’s Data Hypervisor: Your Path to Unified Data
Selector recognized early on that before AI could revolutionize network operations, enterprises first needed a more innovative, more unified way to handle their data. That’s why Selector built its unique Data Hypervisor technology – an innovative approach that transforms the way organizations ingest, enrich, and leverage network, infrastructure, and application data across all seven layers of the stack.
Much like a virtualization hypervisor decouples physical hardware from the virtual machines, Selector’s Data Hypervisor decouples your diverse data sources from their native formats. The hypervisor ingests every type of operational data imaginable – logs, metrics, events, configurations, operational states, and flow data from networks, infrastructure, and applications – then automatically normalizes and enriches this data to provide a unified, vendor-agnostic view. This data normalization makes previously siloed data streams ready for advanced analytics and unified dashboards, thereby eliminating the need for costly manual correlation.
But normalization is only part of the story. The Data Hypervisor also enriches incoming data with critical contextual metadata, such as labels indicating location, peer devices, circuit IDs, customer name, or application relationships, making the data more meaningful. Context transforms isolated events into actionable insights, bridging the gaps between siloed tools and datasets.
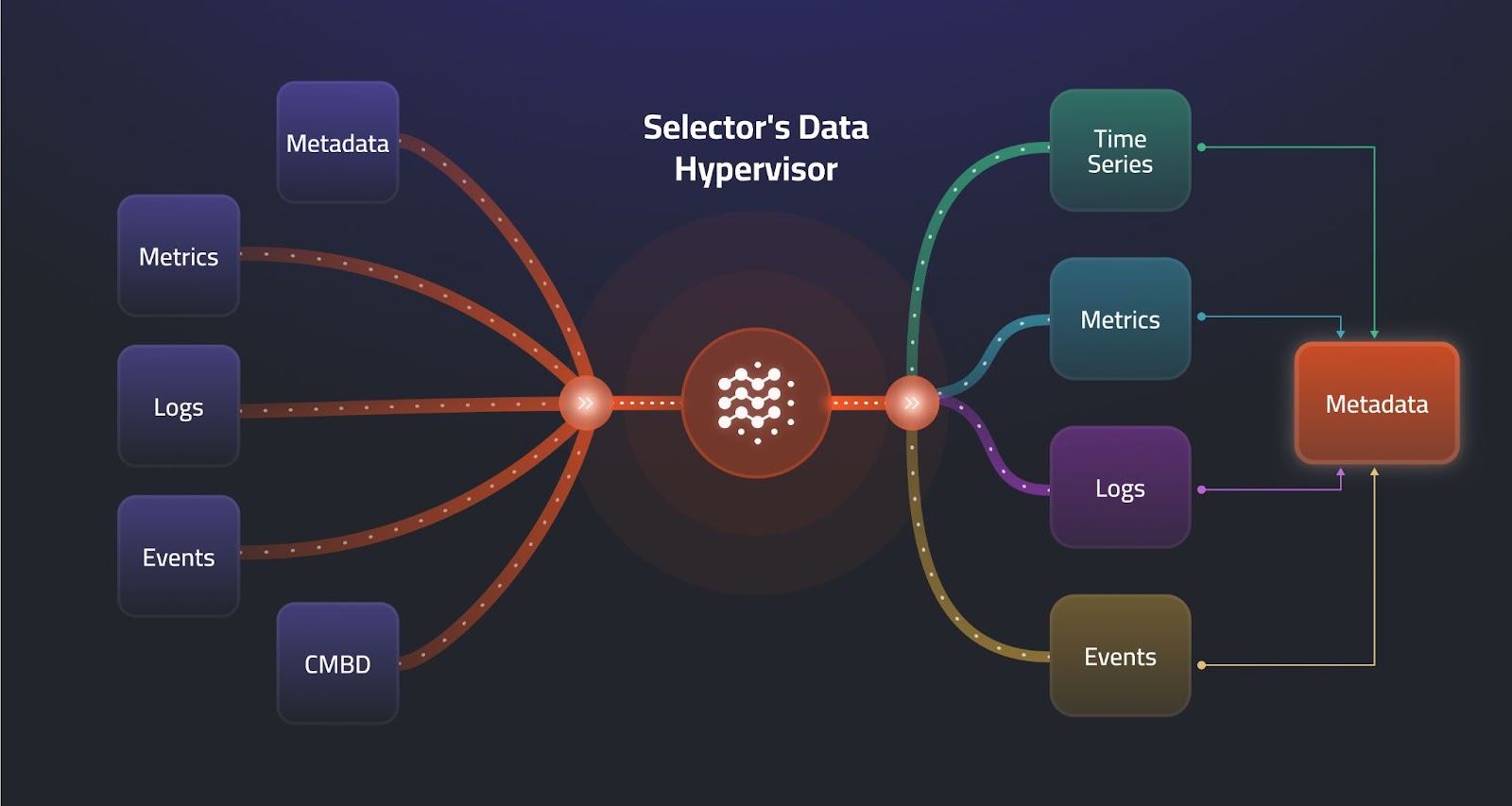
How Selector Uses Machine Learning to Automate Data Enrichment
Traditional methods for parsing and enriching data often depend on rigid rules and manually maintained regular expressions. This is a fragile, maintenance-intensive approach. Selector’s Data Hypervisor replaces these outdated methods with advanced machine learning models that automatically interpret and structure unstructured or semi-structured data.
Rather than needing thousands of handcrafted parsing rules, Selector’s ML-driven approach quickly and accurately extracts relevant information, categorizes events, identifies anomalies, and clusters related issues. This capability drastically reduces manual overhead and error rates, enabling IT teams to shift their focus from managing data to solving actual problems.
This isn’t just theoretical: Selector customers consistently achieve drastic reductions in alert noise – up to a 98% reduction in ticket volume – enabling teams to focus immediately on real issues.
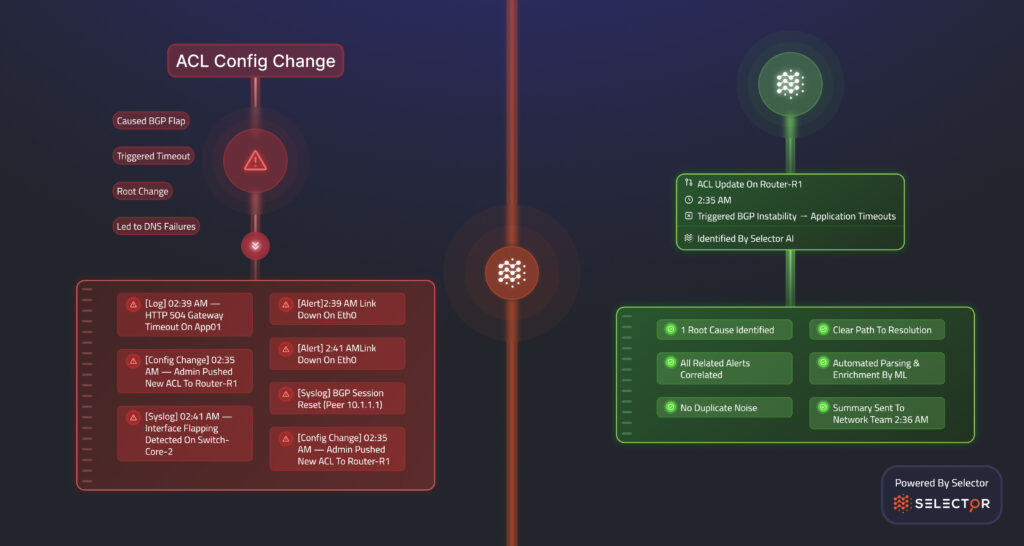
Laying the Foundation for AI-Driven Insights
Selector’s approach to data harmonization is more than just operational convenience. It is essential groundwork for full-stack, AI-driven network operations. Studies in machine learning research emphasize that raw data preprocessing is a challenging and time-consuming task that directly impacts model performance, with a substantial portion of raw data requiring transformation before it becomes useful for AI applications. Selector’s meticulous data enrichment and normalization significantly enhance the usability of data collected from all layers, ensuring that the resulting insights and predictions are accurate, actionable, and trustworthy.
Furthermore, Selector’s solution delivers immediate value. Unlike traditional approaches that require months of extensive setup, Selector can begin providing insights within days, without the need for massive infrastructure investments, such as GPUs. This rapid time-to-value, combined with cost efficiency, makes Selector not only powerful but also practical for businesses looking to make AI-driven operations a reality.
What’s Next: From Unified Data to Autonomous Networks
Effective AIOps isn’t just about adopting AI tools, but also about thoughtfully preparing your infrastructure to support them. Selector’s Data Hypervisor clears away the chaos, laying a robust foundation for next-level AI applications, such as automated correlation, natural language querying, conversational interfaces, and autonomous network operations.
In our next blog, we’ll explore how Selector leverages machine learning to correlate network events in real time, unlocking automated insights and laying the groundwork for predictive analytics and AI-driven automation.
Ready to transform your network operations? Schedule a demo today to see Selector in action, and follow us on LinkedIn or X to be notified of the next blog in this series as we continue your journey toward autonomous network management.